Short article about Web3 - what it is and why I personally think that for the next 3 years it will be must know / must use / must to be in !!
My plan is to write 5 articles:
1. Intro: Web 1.. 2.. 3.. [this one]
2. Layers in crypto.
3. Applications - not only DeFi!
4. Decentralisation
5. Summary - where we are, where to look, why should we join
Web3 is an idea for a new iteration of the World Wide Web-based on the blockchain, which incorporates concepts including decentralization and token-based economics. Some technologists and journalists have contrasted it with Web 2.0, wherein they say data and content are centralized in a small group of companies sometimes referred to as "Big Tech".
Web 1.0 and Web 2.0 refer to eras in the history of the World Wide Web as it evolved through various technologies and formats. Web 1.0 refers roughly to the period from 1991 to 2004, where most websites were static web pages, and the vast majority of users were consumers, not producers, of content.
Web 2.0 is based around the idea of "the web as platform" and centers on user-created content uploaded to social media and networking services, blogs, and wikis, among other services. Web 2.0 is generally considered to have begun around 2004 and continues to the current day.
Visions for Web3 differ, but they revolve around the idea of decentralization and often incorporate blockchain technologies, such as various cryptocurrencies and non-fungible tokens (NFTs). Bloomberg has described Web3 as an idea that "would build financial assets, in the form of tokens, into the inner workings of almost anything you do online". Some visions are based on the concept of decentralized autonomous organizations (DAOs). Decentralized finance (DeFi) is another key concept; in it, users exchange currency without bank or government involvement. Self-sovereign identity allows users to identify themselves without relying on an authentication system such as OAuth, in which a trusted party has to be reached in order to assess identity. Technology scholars have argued that Web3 would likely run in tandem with Web 2.0 sites likely adopting Web3 technologies in order to keep their services relevant.

To believers, Web3 represents the next phase of the internet and, perhaps, of organizing society. Web 1.0, the story goes, was the era of decentralized, open protocols, in which most online activities involved navigating to individual static webpages. Web 2.0, which we’re living through now, is the era of centralization, in which a huge share of communication and commerce takes place on closed platforms owned by a handful of super-powerful corporations—think Google, Facebook, Amazon—subject to the nominal control of centralized government regulators. Web3 is supposed to break the world free of that monopolistic control.
At the most basic level, Web3 refers to a decentralized online ecosystem based on the blockchain. Platforms and apps built on Web3 won’t be owned by a central gatekeeper, but rather by users, who will earn their ownership stake by helping to develop and maintain those services.
Gavin Wood coined the term Web3 (originally Web 3.0) in 2014. At the time, he was fresh off of helping develop Ethereum, the cryptocurrency that is second only to Bitcoin in prominence and market size. Today he runs the Web3 Foundation, which supports decentralized technology projects, as well as Parity Technologies, a company focused on building blockchain infrastructure for Web3. Wood, who is based in Switzerland, spoke with me last week over a video about where Web 2.0 went wrong, his vision of the future, and why we all need to be less trusting. The following interview is a transcript of our conversation, lightly edited for clarity and length.
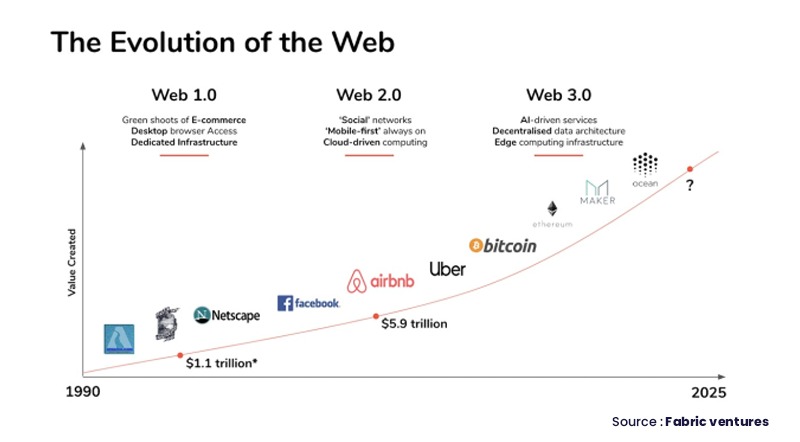
As technology continues to take center stage as a key differentiator for companies, new tech trends for 2022 are beginning to emerge. These trends are largely reflective of the changing realities around us. Successive global lockdowns have opened up a world of possibilities around virtual experiences and digital interactions. The increased urgency around resource scarcity, both human and natural, has also led to the introduction of technology geared towards efficiency and sustainability. These growing trends are part of a larger directional shift of the world wide web to Web 3.0.
To understand the concept of Web 3.0, we need to take a step back and understand the larger evolution of the internet that brought us to this point.
Web 1.0, the original conception of the internet, was a largely static, one-to-many format where users could view web pages but do little beyond that.
Web 2.0 introduced the concept of a worldwide community of internet users, encouraging them to form social media groups, interact with each other and create virtual experiences. Web 2.0, the current form of the internet, is still by large the most influential, but Web 3.0 is now slowly coming into its own.
Web 3.0 takes the notion of the ‘community and expands it to include community ownership and regulation of the internet as a whole. Three key philosophies are involved in web 3.0 definition:
The internet is ‘open’ and is built with open-source software that anyone can create, utilize and modify.
Interactions between users are not governed by a trusted third-party regulatory body.
Anyone can participate, without requiring permission from governments or regulatory bodies.
We can already see many manifestations of web 3.0 all around us. Cloud technology and artificial intelligence are some of the most prevalent forms of web 3.0 today.
In 2022, we will become increasingly familiar with the concept of a “metaverse” – persistent digital worlds that exist in parallel with the physical world we live in – Forbes
Here are **a few of the top technological trends of 2022** that web 3.0 has given rise to.
1. Advanced applications of Artificial Intelligence
Artificial Intelligence has arguably been one of the biggest technological innovations of our time. As AI begins to get more sophisticated and closely mimic human intelligence, a new form will gain popularity in 2022: generative AI. Unlike traditional AI models that simply understand repetitive patterns and recreate them, generative AI is capable of producing completely new material. It uses the underlying principle of AI, learning patterns, to identify how input is linked together and uses it to create new content from code, images, text or video inputs.
One of the biggest applications of generative AI is in customer service. Chatbots of 2022 could be so human-like that it would be practically impossible to differentiate between an AI chatbot and a human rep. AI could also change the way we consume content. Social media algorithms could get a lot smarter with AI and offer more accurate content recommendations. This could deliver even more personalized experiences for customers.
2. Low-code application building software
Creating a strong digital presence has become increasingly important for businesses, especially in the wake of the lockdowns. Mobile applications are one of the most important virtual assets a business can have because it’s the closest way to mimic an in-store experience. Historically, however, applications have been expensive to develop. Businesses needed to either have an in-house software development team or enough financial budgets to outsource the project to a vendor. These have acted as strong barriers to entry and have meant that small businesses could not afford to launch a mobile app. But with web 3.0, a number of low-code or no-code app development platforms have begun to sprout. These platforms make it extremely easy to develop an app with little to no coding knowledge required. Usually, they come with preset templates and features and users have to simply drag and drop them to build their app. These services will undoubtedly democratize the development of mobile applications and help businesses engage with their customers online.
3. Dominance of cloud technology trends
The cloud was one of the biggest game-changing technologies of 2021 as it enabled businesses to work remotely, from any part of the world. But even as offices begin to re-open, it’s unlikely that we’re ever going to see a shift to old on-premise models again. Global scenarios are unpredictable and businesses need to remain agile. As companies plan for this volatile future, they are more likely to move away from hastily implemented cloud setups to cloud-native platforms.
The ‘lift and shift’ approach involved using the same organizational processes and simply moving them to the cloud. This approach might have worked as a time-sensitive solution, but they don’t deliver the full benefits of the cloud in the long term. A major focus for CIOs in the coming year will be implementing more sustainable cloud structures that are scalable and flexible.
One study by Gartner estimates that as much as 95% of new digital initiatives in 2022 will be built upon cloud-native platforms. This underscores the importance of cloud technology in the coming year.
4. Secure data fabric
In line with creating flexible and accessible platforms, data fabric is a type of data architecture that ties together different platforms and users. It’s the antidote to data silos of the past that would often result in a loss of critical insights and make access extremely restricted. A data fabric promises greater efficiency and security because data is stored on a secure cloud platform. This minimizes the cost of storing data and ensures the highest level of encryption.
Think of a data fabric like a self-driving car. When the driver is active then the autonomous mode takes a back seat. If the driver gets lazy and is not too alert then the semi-autonomous mode kicks in and makes course corrections, This is similar to how a data fabric works. It will monitor all data pipelines as a passive observer and once it understands, it will start to suggest more productive alternatives. For instance, a supply chain organization that uses data fabric can keep adding newly encountered data information that can improve decisions based on the newly integrated data.
To implement data fabric design, new technologies like semantic knowledge graphs, embedded machine learning etc. will be needed.
5. Growth of 5G technology
5G technology will ultimately be the backbone that drives all of the above technology trends. 5G networks have a much wider reach than 4G, offer faster internet speeds and even enable heavy code to download seamlessly. 2022 will most likely see 5G enter the mainstream market and become the most widespread form of mobile broadband connectivity. This presents a huge opportunity for businesses. As the new year quickly approaches, CIOs should brainstorm new ways to present their business digitally and new channels that they can open up, powered by 5G technology.
Technology is constantly evolving and these 5 tech trends are just a few of the many we expect to see develop over the next year. As the very form of the internet evolves with the introduction of web 3.0, there’s only one thing that’s for certain: businesses need to respond positively to change and leverage the latest technological advances if they want to maintain their competitive edge.
Web 2 was boom - lots of new companies emerged, Web 3 will go into the same direction with a possibility to be a much bigger change to our way of living!